
Generative AI is changing the way online information is surfaced, with platforms like ChatGPT, Google's Gemini (AI Overviews), and Perplexity offering synthesized answers that cite only a handful of sources. Over 12 weeks, we tracked which content types these AI engines referenced most across various query types. Below is a concise summary of the findings. This is a follow up to our previous study about what sources to AI search engines cite to help marketers improve AI search visibility.
Table of contents:
- What content do LLMs use the most?
- What content is cited for B2B vs B2C?
- How do citations differ between different regions?
- How does content type change across different parts of the funnel?
- Key Takeaways
1. What content do LLMs use the most?
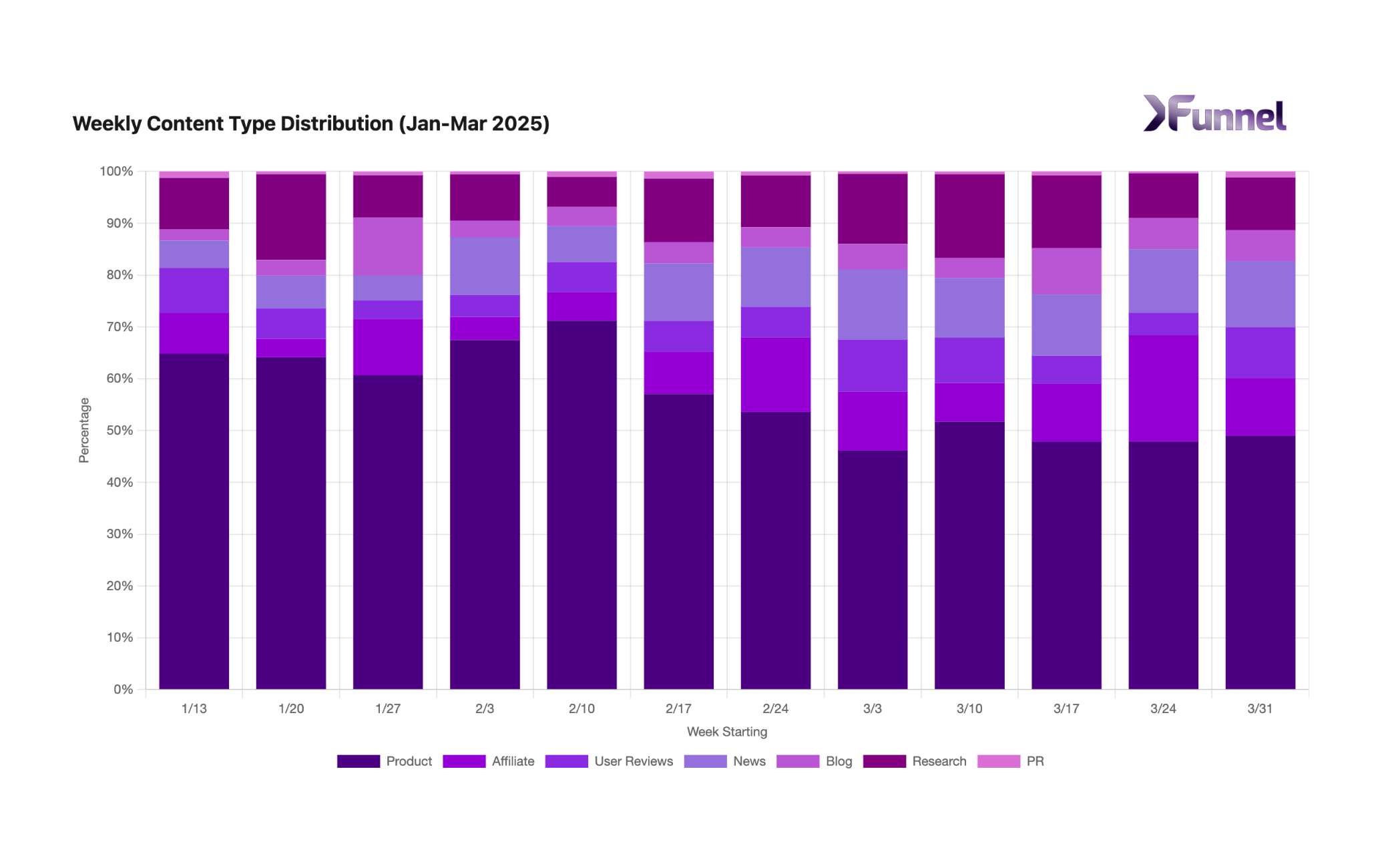
Over each of the 12 weeks, Product-related content (including "best of" articles, vendor comparisons, head to head comparisons, and product pages directly from vendors) dominated AI citations, ranging from roughly 46% to over 70% of all cited sources. This preference appears consistent with how AI engines handle factual or technical questions, using official pages that offer reliable specifications, FAQs, or how-to guides. By comparison:
-
News and Research each occupied around 5–16% of citations, depending on the week. News provided timely context, while research (including academic papers or whitepapers) offered authoritative insights for more in-depth or scientific topics.
-
Affiliate content typically stayed in single-digit percentages, except for one spike above 20%. This suggests occasional AI reliance on blatantly affiliate style content that doesn't have reliable product related information.
-
User Reviews (forums, Q&A communities, consumer feedback) hovered between 3% and 10%. Perplexity.ai, for instance, sometimes pulls directly from Reddit threads for product queries.
-
Blogs remained a smaller slice in most weeks (about 3–6%), indicating that only a few exceptional blog articles surfaced as prime references.
-
PR content (press releases) barely registered, usually under 2%.
This distribution shows a recurring pattern: official or fact-rich pages rise to the top, while news, research, reviews, and occasional affiliate sites fill specific niches in AI-generated answers.
2. What content is cited for B2B vs B2C?
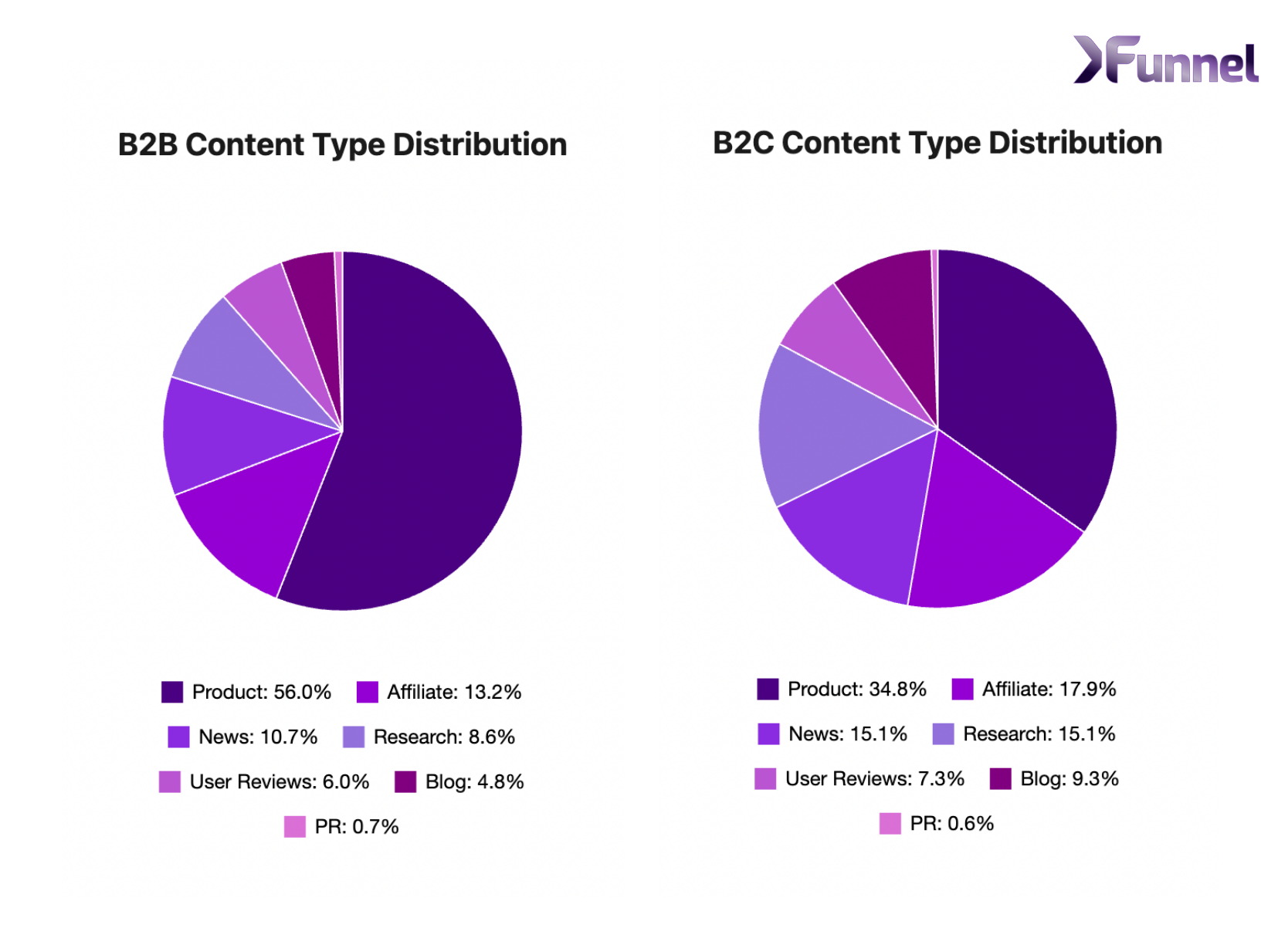
When separated into B2B and B2C queries, the data reveals distinct source preferences:
B2B Queries: Nearly 56% of citations were for product pages (company or vendor sites). There was also moderate representation from affiliate (13%) and user reviews (11%), followed by news (~9%) and research (~6%). This highlights a strong reliance on official, first-party resources in business contexts—particularly for technical or enterprise-level questions.
B2C Queries: Product content fell to about 35%, while affiliate (18%), user reviews (15%), and news (15%) surged. AIs often combine manufacturer details with third-party perspectives when addressing consumer-oriented topics. Perplexity, for example, cites Reddit for gadgets, while Google's AI Overviews might quote recognized review outlets or Q&A forums.
In essence, B2B queries lead to fewer, more authoritative sources; B2C queries produce a broader mix, with more voices from affiliates, review sites, and general media.
3. How do citations differ between different regions?
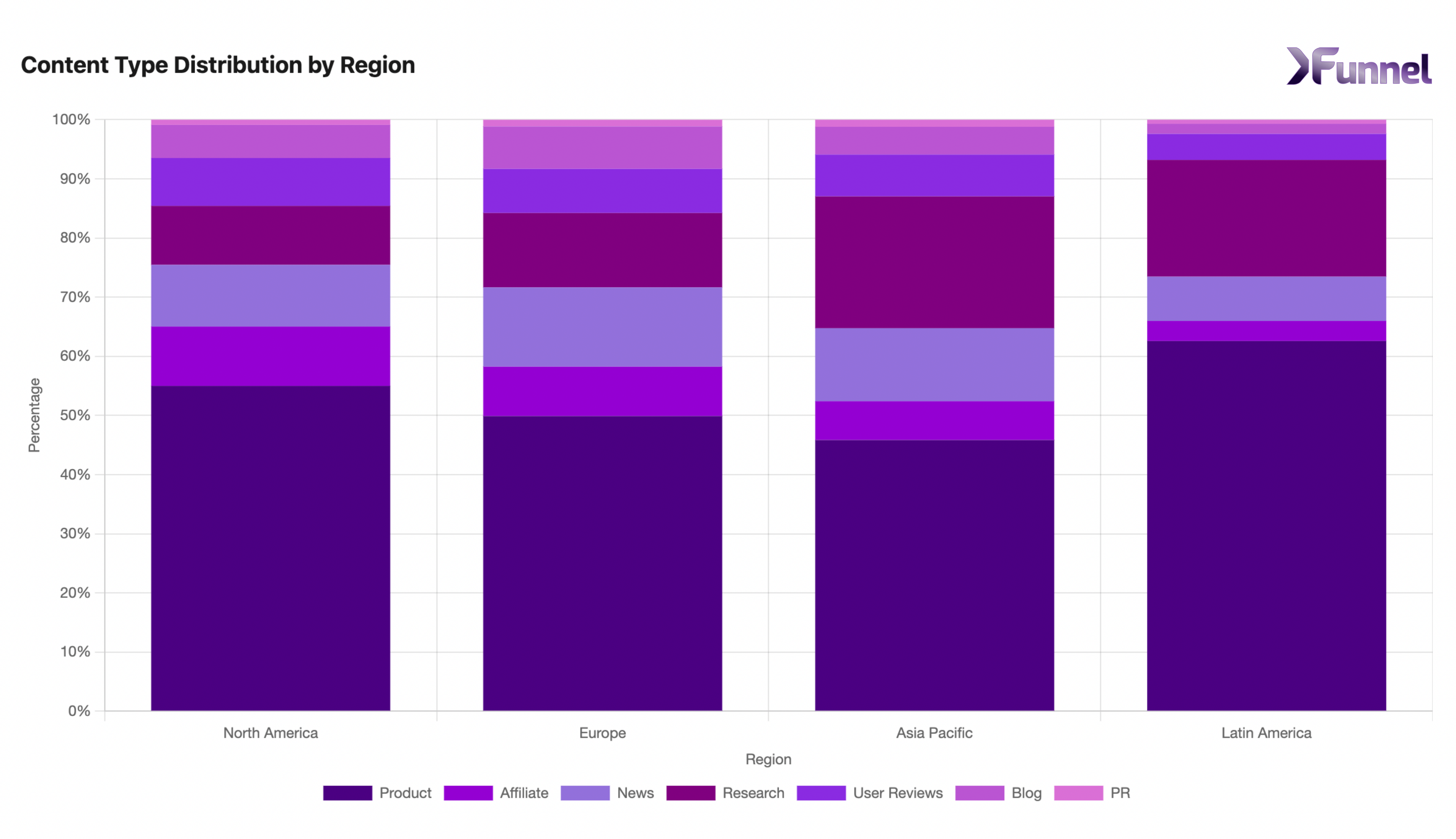
North America (NA): About 55% product citations, with news (~10%) and research (~10%) next in line. The region's rich media and corporate presence likely explains this balance.
Europe: Product references dipped to ~50%, with higher shares for news (13.4%), research (12.6%), and blogs (7.2%). The variety points to a multilingual content ecosystem, where AI engines pull from both official sites and broader media.
Asia Pacific (APAC): Product content fell to 45.9%, while research soared to 22.3%—the highest of any region. AI outputs for APAC queries often defaulted to academic or technical papers, possibly due to fewer local-language alternatives or more science/tech questions.
Latin America: Product citations reached 62.6%, while research also stood out at 19.7%. Affiliate and blog content were each well under 5%. This suggests fewer local third-party sources or a heavier reliance on official product pages and recognized research.
By region, AI engines adapt to the availability and perceived reliability of local content, often preferring either official or research-based sources when other options are less established.
4. How does content type change across different parts of the funnel?
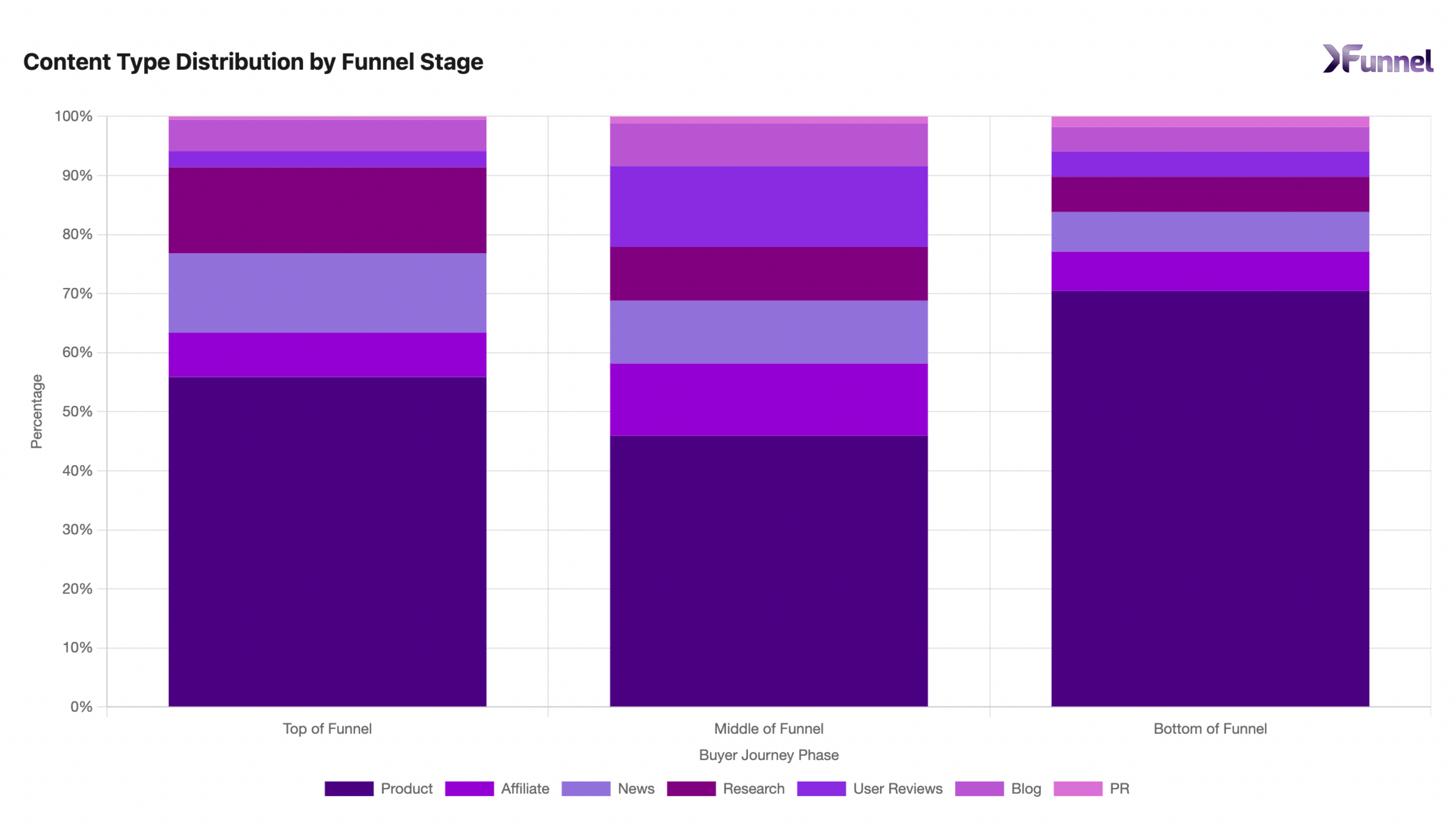
Researchers grouped queries into three broad stages: Top of Funnel (unbranded), Middle of Funnel (branded), and Bottom of Funnel. Each displayed a distinct citation profile.
Top of Funnel (Unbranded: Problem Exploration + Solution Education)
- Product: ~56%
- News & Research: ~13–15% each
- Affiliate & Reviews: Under 10%
Early queries often seek background or broad insights. Official sites can provide educational resources, while news and research offer big-picture perspectives. User reviews rarely appear here, presumably because top-of-funnel questions are more informational than comparative. Marketing teams who want to improve LLM optimization of content should pay attention - as creating top-of-funnel content increases probability of being included in middle-of-funnel content.
Middle of Funnel (Branded: Solution Comparison + User Reviews)
- Product: ~46%
- User Reviews & Affiliate: ~14% each
- News & Blogs: ~10–11% combined
Mid-stage questions about comparing brands or final checks frequently cite third-party evaluations, user forums, and review sites. AI engines gather multiple voices—manufacturer plus community—to address side-by-side comparisons.
Bottom of Funnel (Solution Evaluation)
- Product: 70.46%
- Research, News, Reviews: Mostly single-digit shares
Decision-stage queries focus heavily on specific product details (implementation steps, feature breakdowns, or pricing). AI outputs predominantly cite official documentation or company materials, with minimal reliance on outside commentary.
Key Takeaways
-
Product Content Prevails: Across most weeks, regions, and funnel stages, pages providing facts, specifications, and information about products (vendor comparisons, product pages, best of lists, head to head comparisons, listicles) were very dominant in AI citations. Even unbranded questions featured vendor materials if they provided substantial information.
-
Varied Support Sources: News and research consistently show up as secondary references, particularly when queries involve timely information or scientific depth. We saw news and research matter a lot more in top-of-funnel queries, which makes sense as users are typically problem aware but not solution aware.
-
Distinct B2B vs. B2C Mix: Business-oriented queries rely more on direct company sources, while consumer queries bring in additional user reviews and affiliate coverage.
-
Regional Nuances: AI engines select more research-based content in APAC and rely heavily on product sites in Latin America. North America and Europe show broader content diversity.
-
Funnel Shifts: Early and mid-funnel questions include more external validation (research, news, affiliate, reviews). Bottom-funnel queries tend to cite official product sites almost exclusively.
These observations suggest that large language models prioritize trustworthy, in-depth pages, especially for technical or final-stage information. In consumer scenarios, additional weight is given to reviews and affiliate perspectives, illustrating how generative AI attempts to deliver answers reflecting multiple viewpoints. While the nuances vary by region and query stage, the overarching trend is clear: factually robust, authoritative content remains at the heart of AI-generated citations.
About the author:
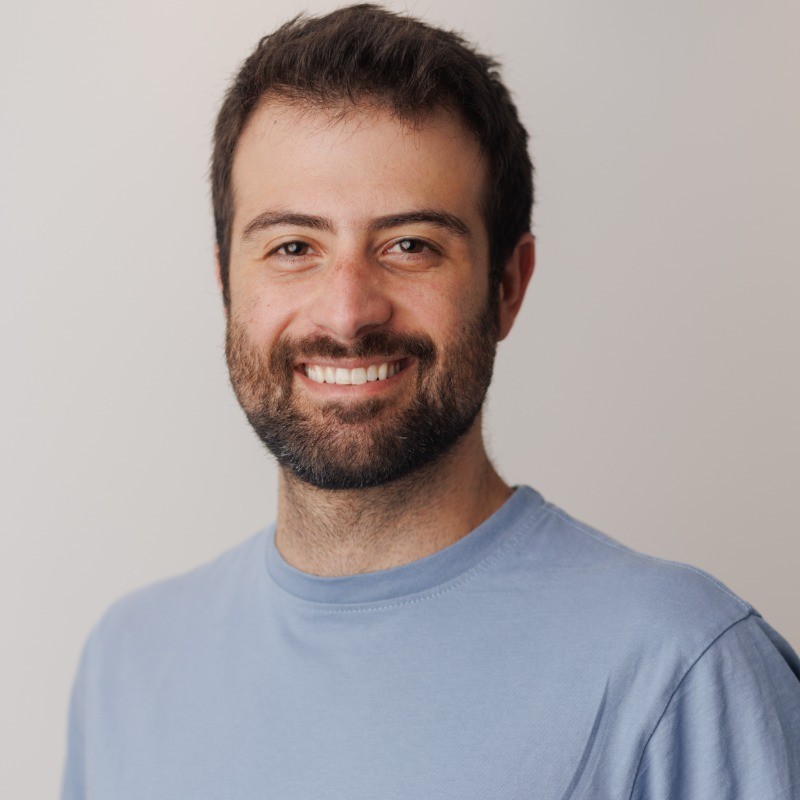
Beeri Amiel is a growth and go-to-market expert who has spent years advising B2B software companies on improving their buying journeys. His deep understanding of how AI-driven search shapes customer discovery led him to develop innovative solutions that bridge the gap between traditional SEO and AI-powered platforms. As the co-founder of xfunnel, Beeri helps businesses optimize their presence across AI search engines.